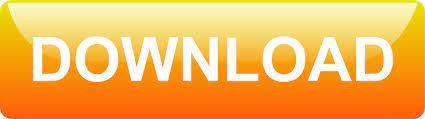
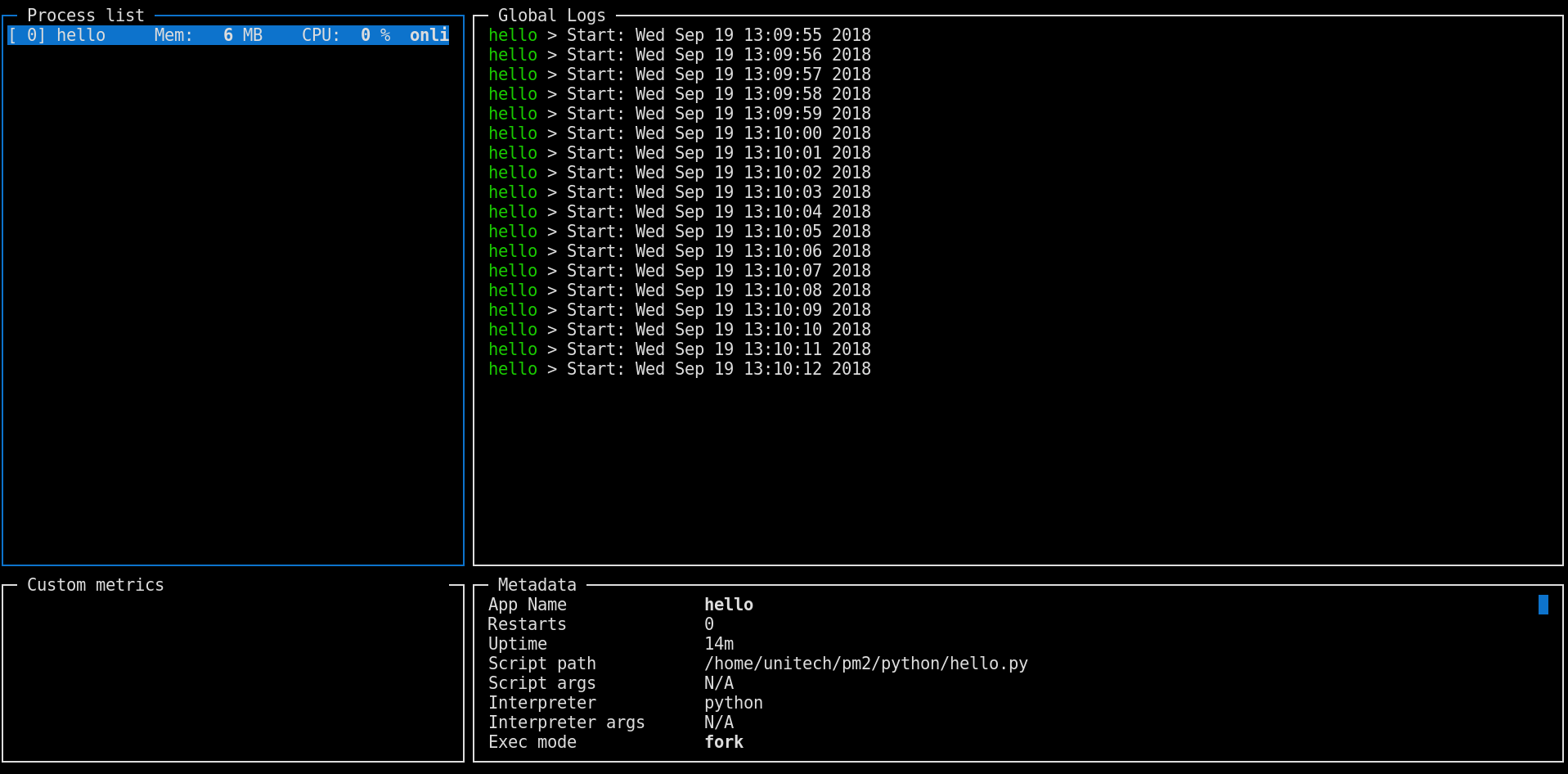
all jobs running on a cluster for a particular user or for more complex deployments, all sub-tasks that together make up a job.Įach of these might become a single target for Borgmon to scrape data from via /varz endpoints, analogous to Prometheus’ /metrics. In its native environment, Borgmon relies on ubiquitous and straightforward service discovery: monitored services are managed by Borg, so it’s easy to find e.g. Prometheus' ancestor and main inspiration is Google's Borgmon. We’ll also discuss why it remains surprisingly involved to get this right. This post describes how we’ve done that in one instance, with a fully worked example of monitoring a simple Flask application running under uWSGI + nginx. Eating the right dogfood here means integrating Prometheus monitoring with our (mostly Python) backend stack. Recently we’ve been working on improving our Prometheus offering. MetricFire allows for on-premises setups, as well as our typical hosted platform, depending on the customer's needs. Our hosted Prometheus solutions retain this flexibility, as we are open-source at heart. MetricFire saves companies money by doing all of that engineering work for them, so they don’t have to hire their own team. Without MetricFire, companies install their own monitoring software and hire engineers to maintain and process that information. Our customers include large multinational coffee brewers, game companies, and other data science/SaaS companies. It also drives the service status transparency our customers love. This has worked out really well for us over the years: as our own customer, we quickly spot issues in our various ingestion, storage and rendering services. We eat lots of our own dogfood at MetricFire, monitoring our services with a dedicated cluster running the same software.
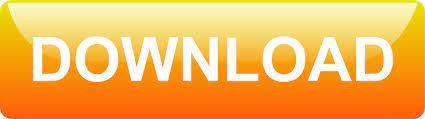